ICA 110381KV7.2/7.3 activator; displays anticonvulsant properties CAS# 325457-99-6 |
- CZC24832
Catalog No.:BCC1507
CAS No.:1159824-67-5
- PI3Kγ inhibitor 1
Catalog No.:BCC4180
CAS No.:1172118-03-4
- PI-103 Hydrochloride
Catalog No.:BCC1860
CAS No.:371935-79-4
- PIK-93
Catalog No.:BCC2519
CAS No.:593960-11-3
- TG100-115
Catalog No.:BCC1247
CAS No.:677297-51-7
- BKM120
Catalog No.:BCC1279
CAS No.:944396-07-0
Quality Control & MSDS
Number of papers citing our products
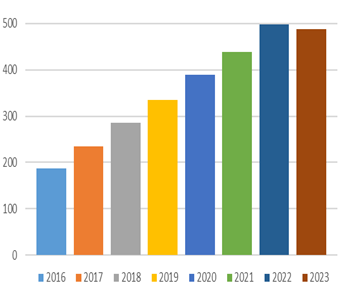
Chemical structure
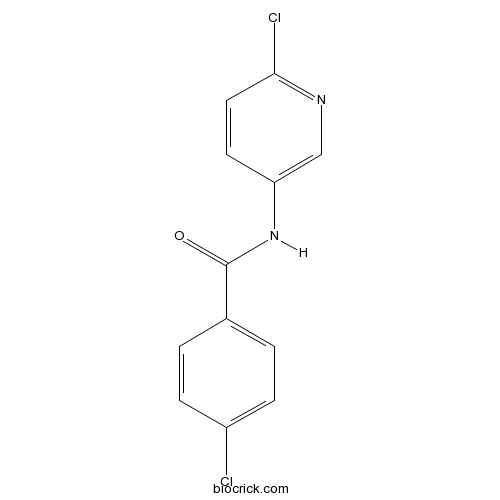
3D structure
Cas No. | 325457-99-6 | SDF | Download SDF |
PubChem ID | 4314115 | Appearance | Powder |
Formula | C12H8Cl2N2O | M.Wt | 267.11 |
Type of Compound | N/A | Storage | Desiccate at -20°C |
Solubility | Soluble to 100 mM in DMSO and to 20 mM in ethanol | ||
Chemical Name | 4-chloro-N-(6-chloropyridin-3-yl)benzamide | ||
SMILES | C1=CC(=CC=C1C(=O)NC2=CN=C(C=C2)Cl)Cl | ||
Standard InChIKey | FOYOGIUAMYSVIT-UHFFFAOYSA-N | ||
Standard InChI | InChI=1S/C12H8Cl2N2O/c13-9-3-1-8(2-4-9)12(17)16-10-5-6-11(14)15-7-10/h1-7H,(H,16,17) | ||
General tips | For obtaining a higher solubility , please warm the tube at 37 ℃ and shake it in the ultrasonic bath for a while.Stock solution can be stored below -20℃ for several months. We recommend that you prepare and use the solution on the same day. However, if the test schedule requires, the stock solutions can be prepared in advance, and the stock solution must be sealed and stored below -20℃. In general, the stock solution can be kept for several months. Before use, we recommend that you leave the vial at room temperature for at least an hour before opening it. |
||
About Packaging | 1. The packaging of the product may be reversed during transportation, cause the high purity compounds to adhere to the neck or cap of the vial.Take the vail out of its packaging and shake gently until the compounds fall to the bottom of the vial. 2. For liquid products, please centrifuge at 500xg to gather the liquid to the bottom of the vial. 3. Try to avoid loss or contamination during the experiment. |
||
Shipping Condition | Packaging according to customer requirements(5mg, 10mg, 20mg and more). Ship via FedEx, DHL, UPS, EMS or other couriers with RT, or blue ice upon request. |
Description | KV7.2/7.3 activator (EC50 = 0.38 μM). Decreases neuronal excitability in CA1 hippocampal neurons. Exhibits anticonvulsive properties in amygdala-kindled rats, a model for complex partial seizures. |

ICA 110381 Dilution Calculator

ICA 110381 Molarity Calculator
1 mg | 5 mg | 10 mg | 20 mg | 25 mg | |
1 mM | 3.7438 mL | 18.7189 mL | 37.4378 mL | 74.8755 mL | 93.5944 mL |
5 mM | 0.7488 mL | 3.7438 mL | 7.4876 mL | 14.9751 mL | 18.7189 mL |
10 mM | 0.3744 mL | 1.8719 mL | 3.7438 mL | 7.4876 mL | 9.3594 mL |
50 mM | 0.0749 mL | 0.3744 mL | 0.7488 mL | 1.4975 mL | 1.8719 mL |
100 mM | 0.0374 mL | 0.1872 mL | 0.3744 mL | 0.7488 mL | 0.9359 mL |
* Note: If you are in the process of experiment, it's necessary to make the dilution ratios of the samples. The dilution data above is only for reference. Normally, it's can get a better solubility within lower of Concentrations. |
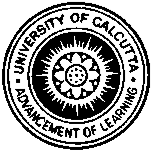
Calcutta University
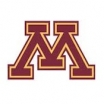
University of Minnesota
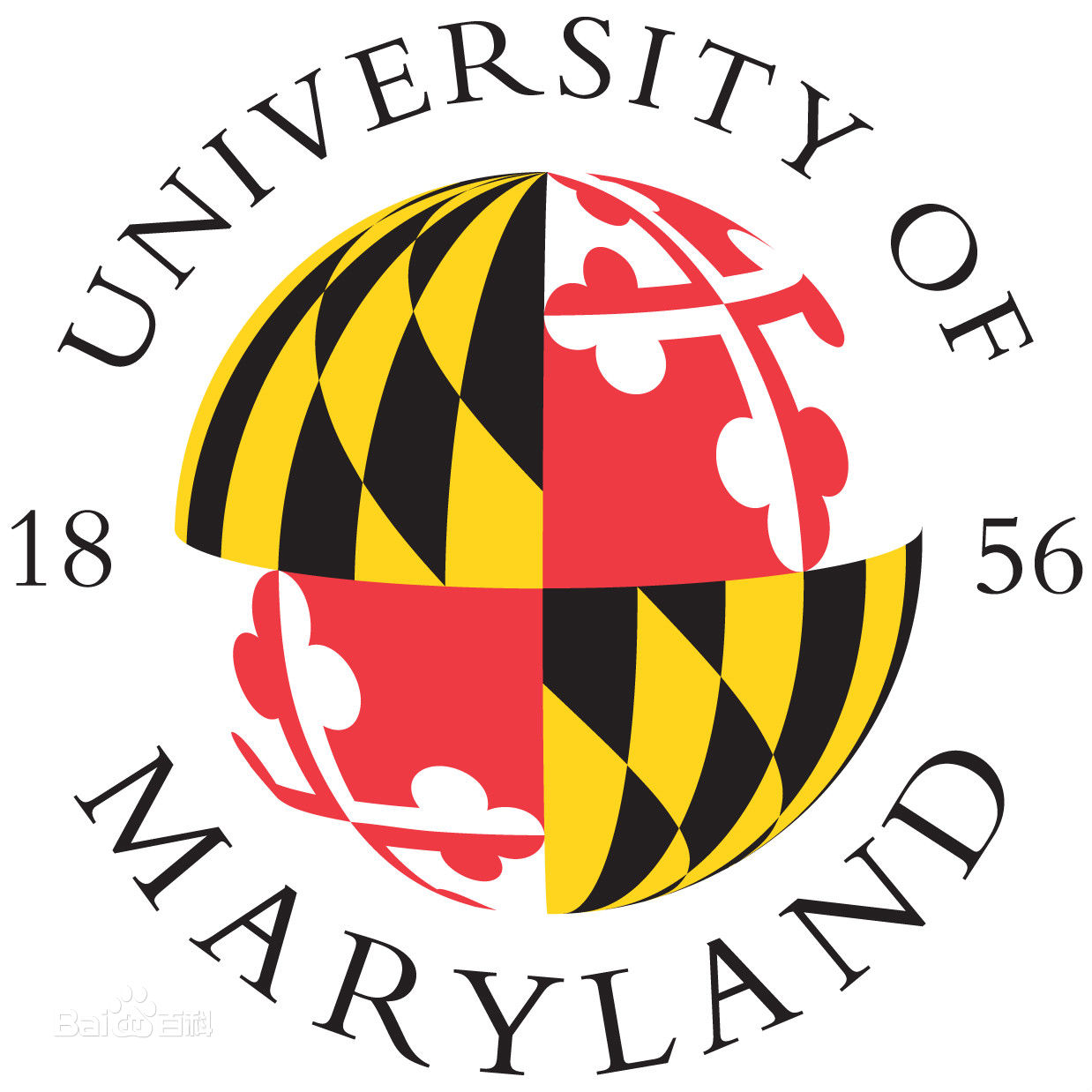
University of Maryland School of Medicine
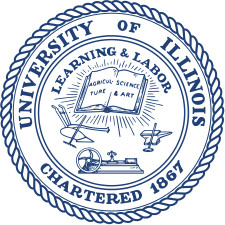
University of Illinois at Chicago
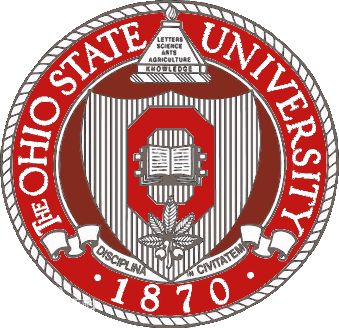
The Ohio State University
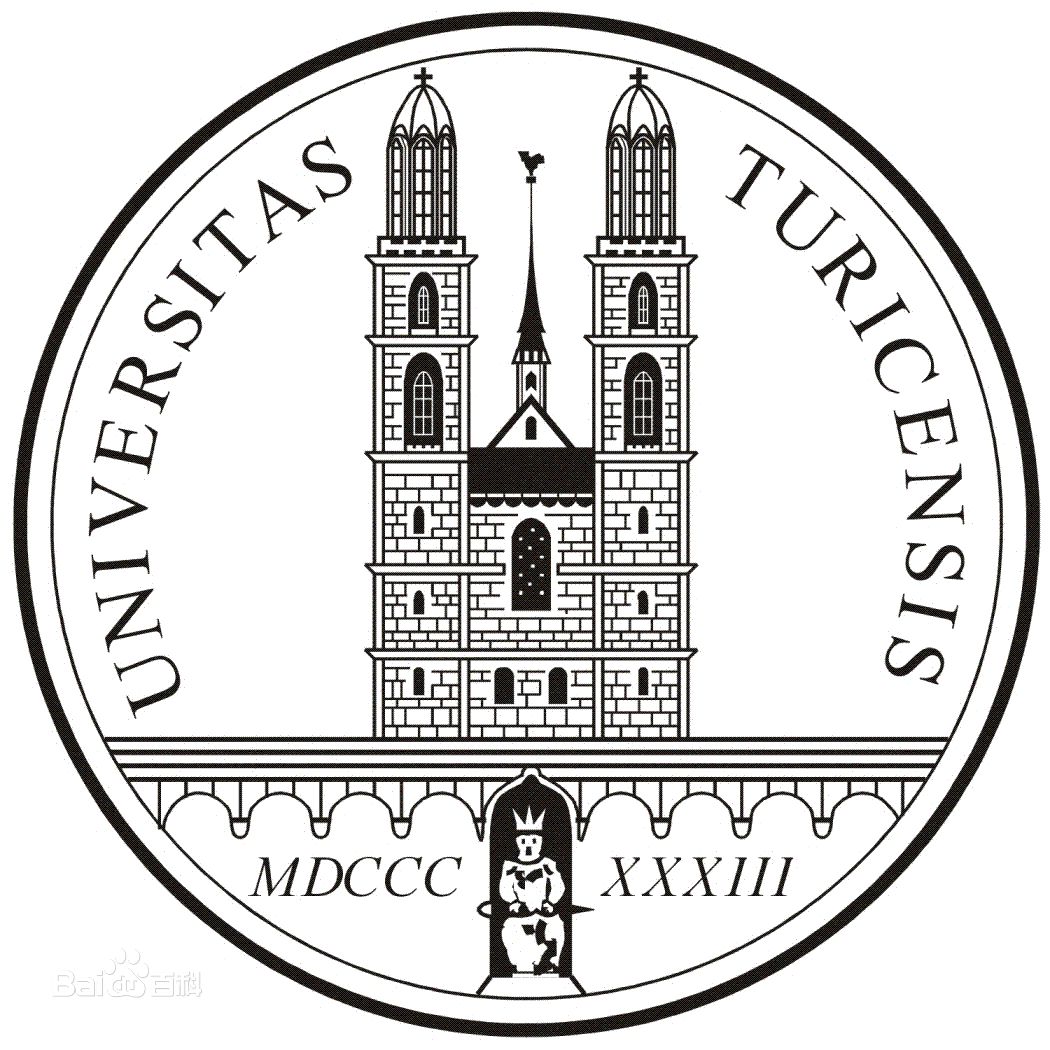
University of Zurich
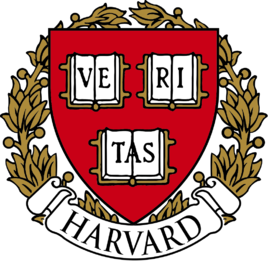
Harvard University
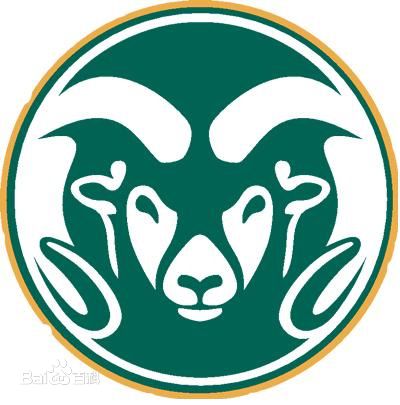
Colorado State University
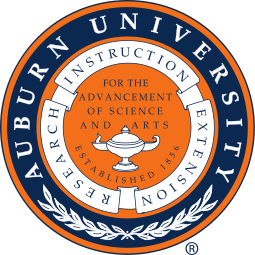
Auburn University
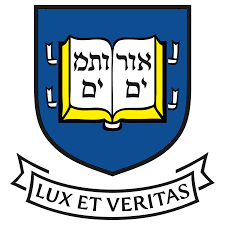
Yale University
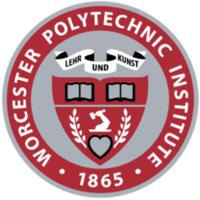
Worcester Polytechnic Institute
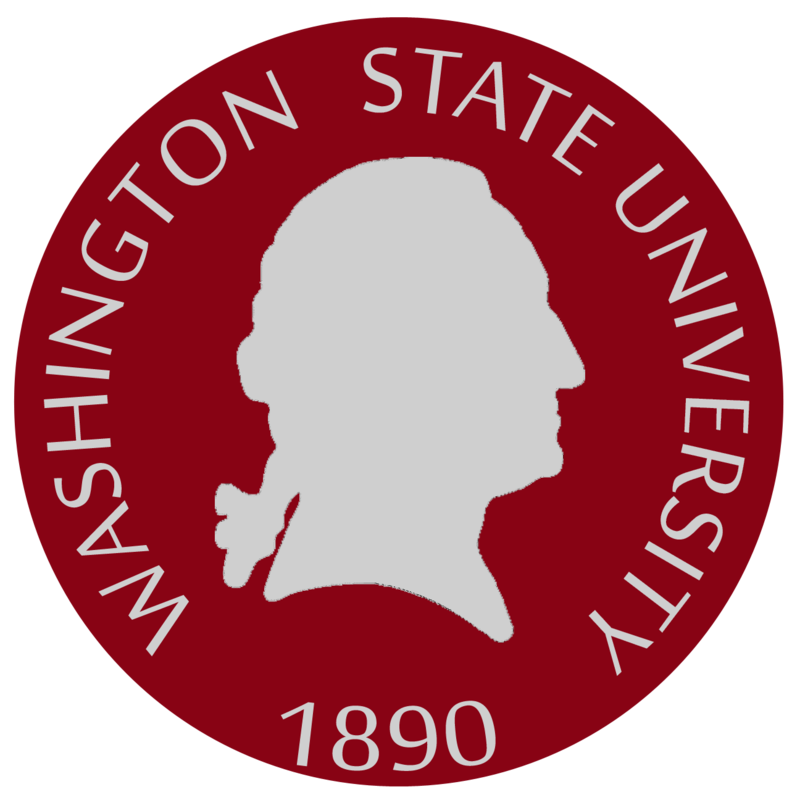
Washington State University
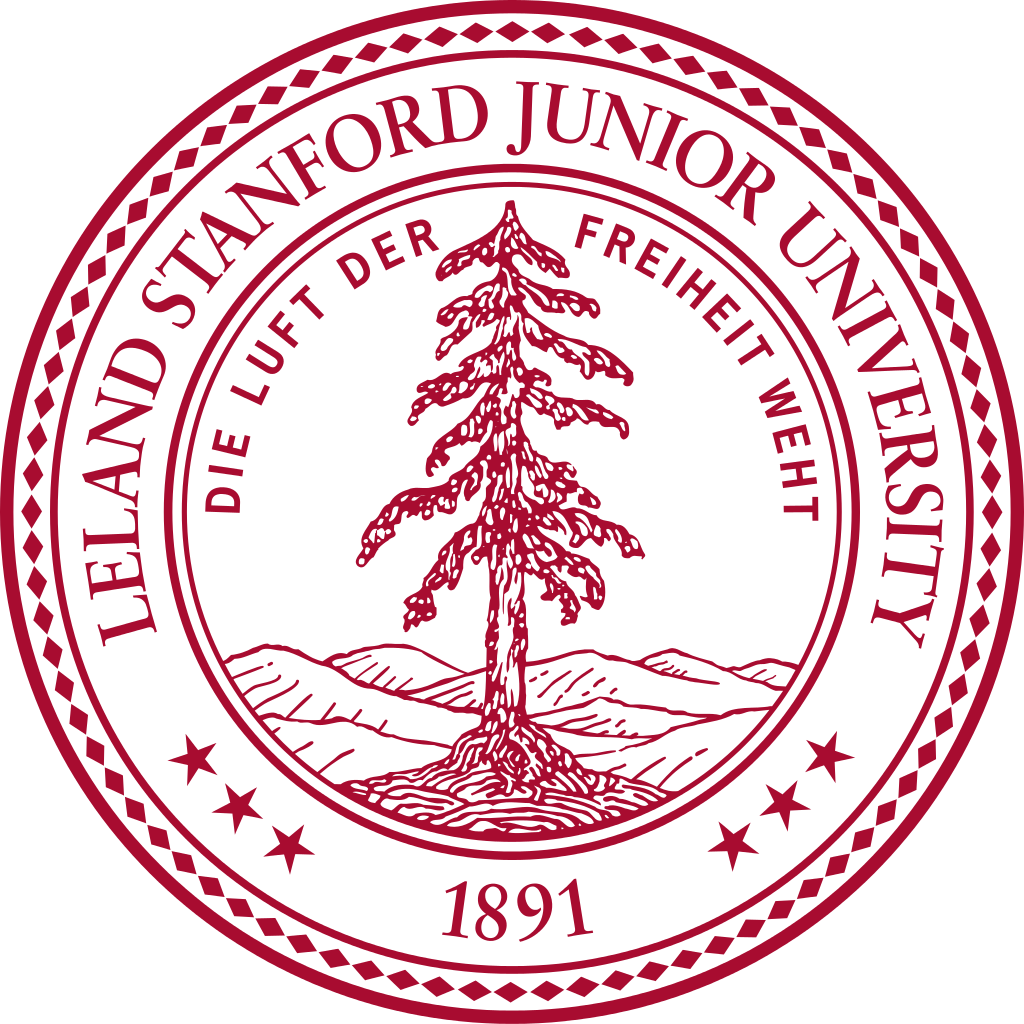
Stanford University
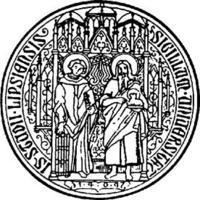
University of Leipzig
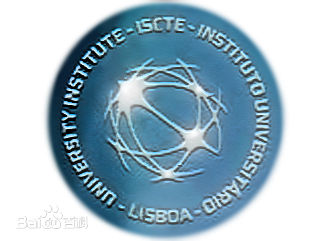
Universidade da Beira Interior
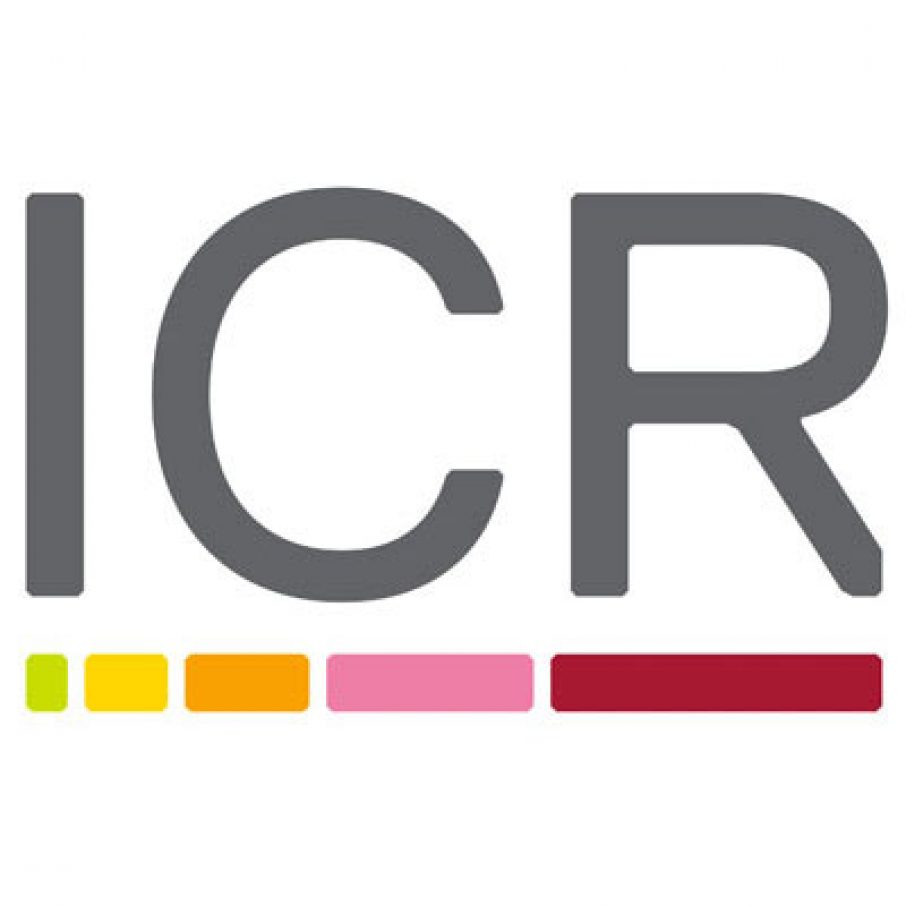
The Institute of Cancer Research
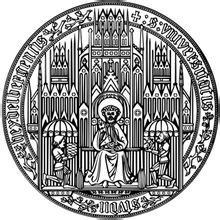
Heidelberg University
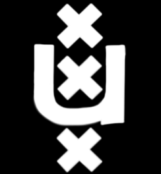
University of Amsterdam
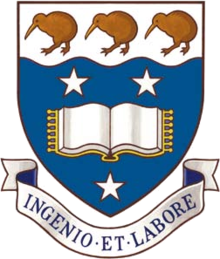
University of Auckland
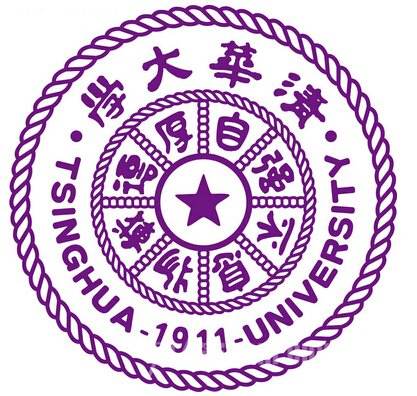
TsingHua University
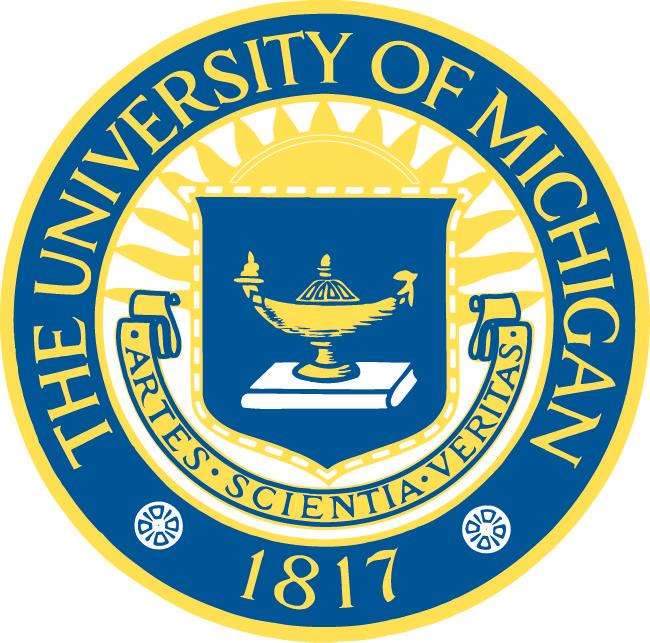
The University of Michigan
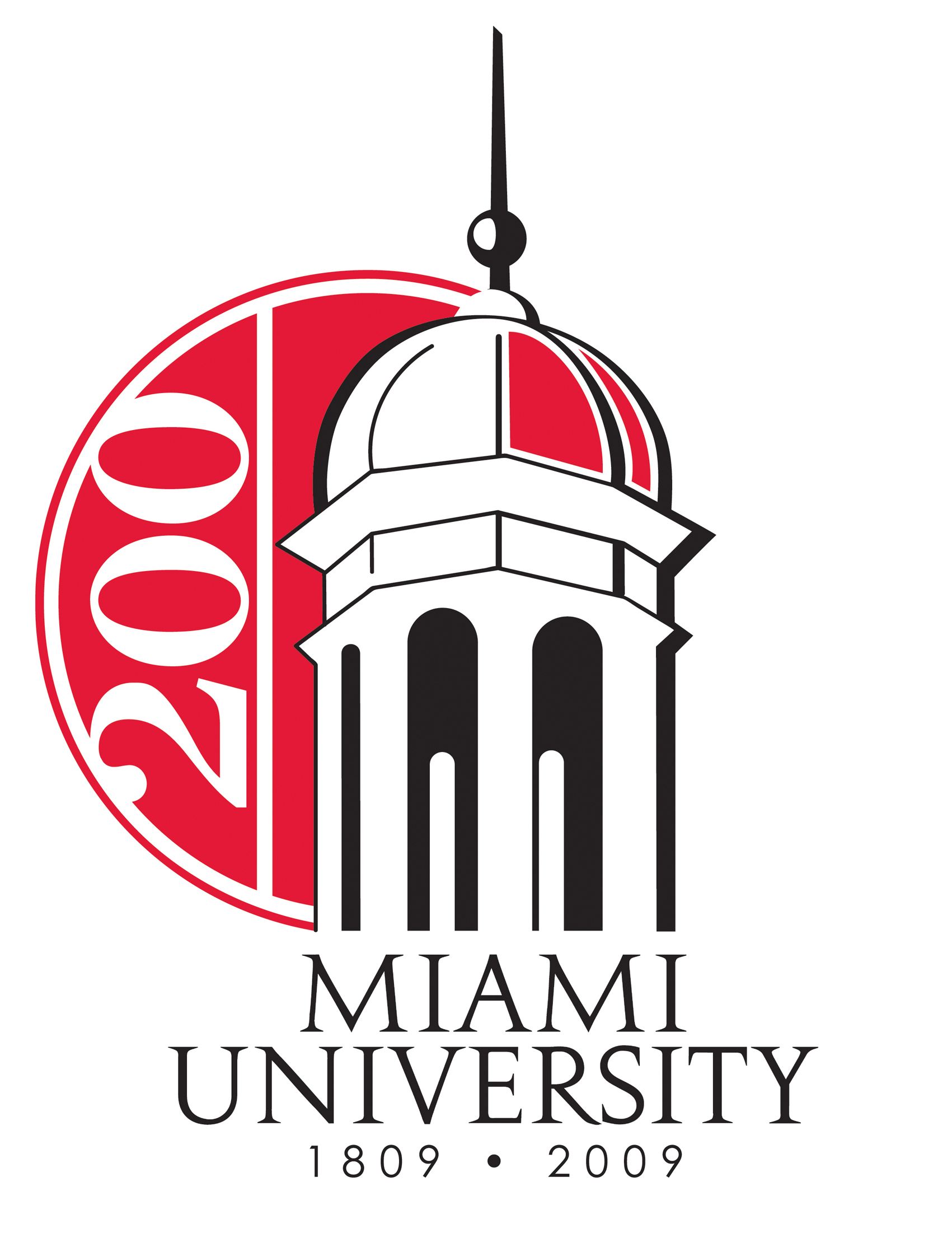
Miami University
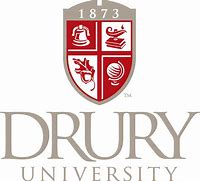
DRURY University
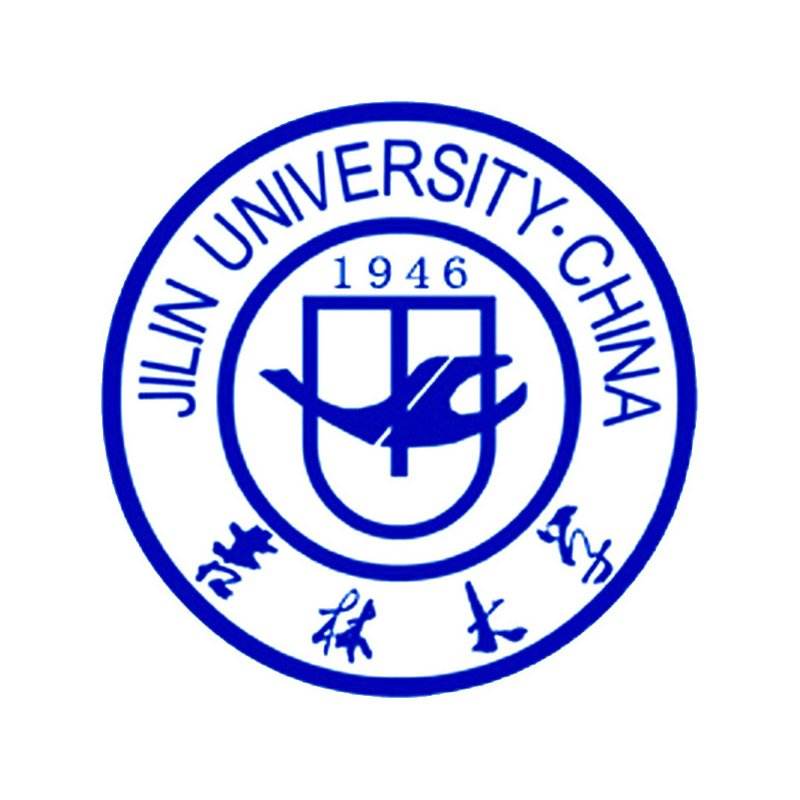
Jilin University
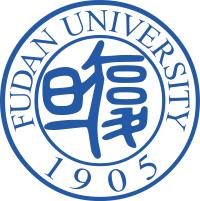
Fudan University
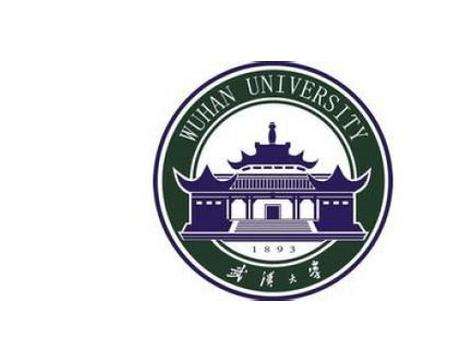
Wuhan University
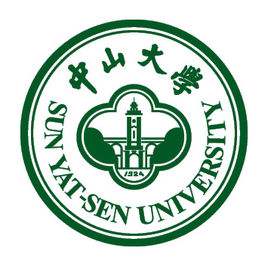
Sun Yat-sen University
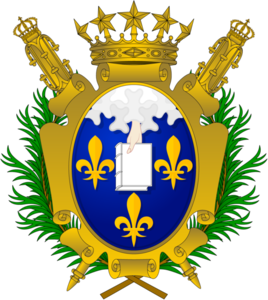
Universite de Paris
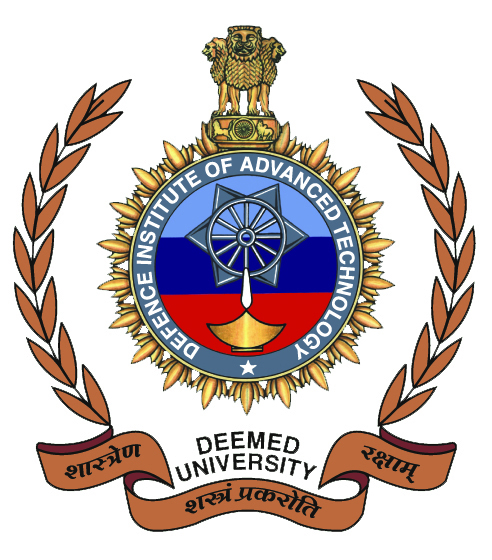
Deemed University
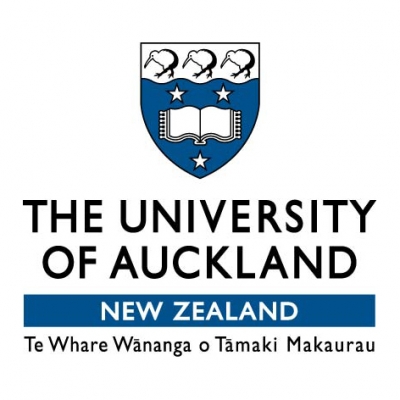
Auckland University
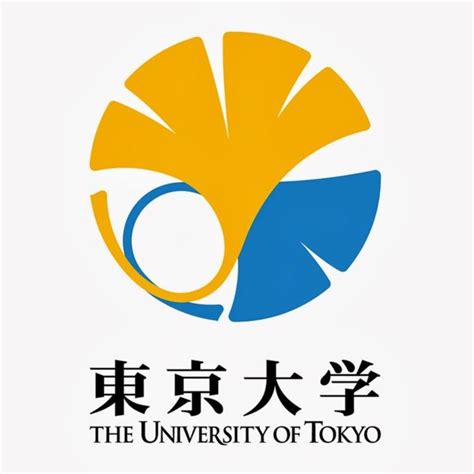
The University of Tokyo
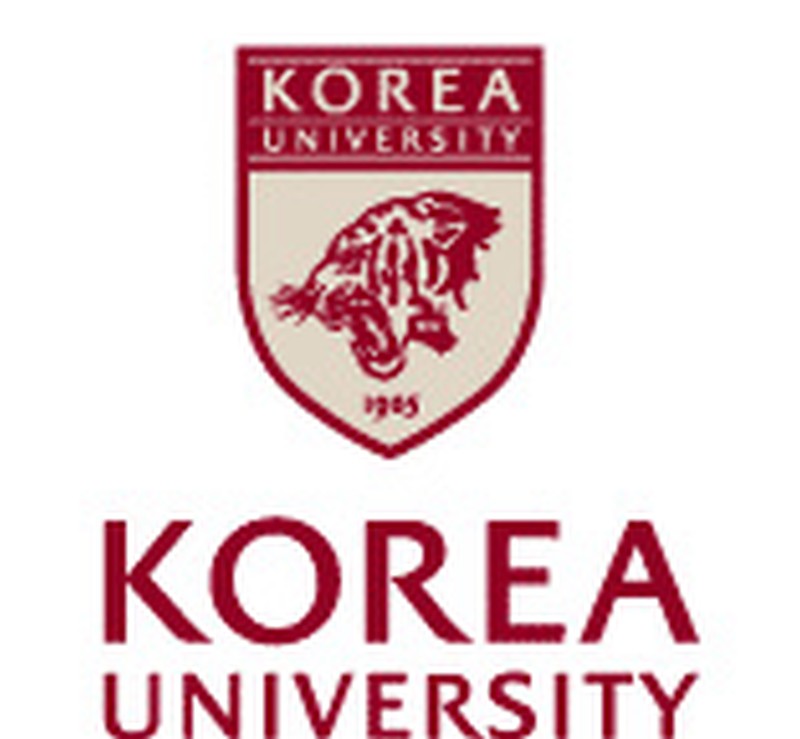
Korea University
- Sulfo-NHS-SS-Biotin
Catalog No.:BCC3580
CAS No.:325143-98-4
- Ergosta-7,22-dien-3-one
Catalog No.:BCN7088
CAS No.:32507-77-0
- Isorhapotogenin
Catalog No.:BCN3383
CAS No.:32507-66-7
- Myricanone
Catalog No.:BCN5243
CAS No.:32492-74-3
- Periplocymarin
Catalog No.:BCN8485
CAS No.:32476-67-8
- T 0156 hydrochloride
Catalog No.:BCC5803
CAS No.:324572-93-2
- 1-Indanamine hydrochloride
Catalog No.:BCC8467
CAS No.:32457-23-1
- NPS-2143 hydrochloride
Catalog No.:BCC1808
CAS No.:324523-20-8
- Isochlorogenic acid
Catalog No.:BCN5910
CAS No.:534-61-2
- 20(29)-Lupene-3,23-diol
Catalog No.:BCN5242
CAS No.:32451-85-7
- Khasianine
Catalog No.:BCN2530
CAS No.:32449-98-2
- H-D-Cys-OH.H2O.HCl
Catalog No.:BCC2913
CAS No.:32443-99-5
- 1-Oleoyl lysophosphatidic acid sodium salt
Catalog No.:BCC7792
CAS No.:325465-93-8
- Ascleposide E
Catalog No.:BCN5244
CAS No.:325686-49-5
- Indiplon
Catalog No.:BCC7720
CAS No.:325715-02-4
- BPIPP
Catalog No.:BCC7730
CAS No.:325746-94-9
- GRI 977143
Catalog No.:BCC2401
CAS No.:325850-81-5
- Kaempferol 3-neohesperidoside
Catalog No.:BCN5245
CAS No.:32602-81-6
- Oleuropein
Catalog No.:BCN5246
CAS No.:32619-42-4
- Boc-Ser-OH
Catalog No.:BCC3439
CAS No.:3262-72-4
- Fraxamoside
Catalog No.:BCN5247
CAS No.:326594-34-7
- Mesoridazine Besylate
Catalog No.:BCC3975
CAS No.:32672-69-8
- H-Glu(OtBu)-OtBu.HCl
Catalog No.:BCC2934
CAS No.:32677-01-3
- Edpetiline
Catalog No.:BCN6771
CAS No.:32685-93-1
Deletion of mazF increases Staphylococcus aureus biofilm formation in an ica-dependent manner.[Pubmed:28334216]
Pathog Dis. 2017 Jul 31;75(5). pii: 3063887.
Toxin-antitoxin (TA) systems are composed of a toxin that inhibits an essential cellular process (e.g. DNA replication, transcription, membrane integrity) and its cognate antitoxin that neutralizes the effect of the toxin. Staphylococcus aureus harbors two types of chromosomally encoded TA systems, namely mazEFsa encoding a UACAU-specific mRNA interferase and two paralogous genes of yefM-yoeBsa encoding a ribosome-dependent endoribonuclease system. However, little is known about the physiological role of MazEFsa and YefM-YoeBsa in S. aureus. Upon characterizing the phenotypes of single, double and triple gene deletion mutants, we found that mazFsa deletion led to increased biofilm formation. Subsequently, transcriptional analysis revealed that expression of intercellular adhesin (ica) gene, icaADBC, increased in a mazFsa deletion mutant. mazFsa/icaADBC double gene deletion and genetic complementation approaches provided convincing evidence that increased biofilm formation was caused by an increase in polysaccharide intercellular adhesin synthesized by icaADBC-encoded proteins. Furthermore, through the use of alanine substitutions at the conserved active residues of MazFsa, our results suggested that ica-mediated biofilm formation depended on the mRNA interferase activity of MazFsa. These findings give new insights not only into the physiological role of MazEFsa in S. aureus, but also into the regulatory mechanism of ica-dependent biofilm formation.
Multicomponent quantitative spectroscopic analysis without reference substances based on ICA modelling.[Pubmed:28299416]
Anal Bioanal Chem. 2017 May;409(13):3319-3327.
A fast and reliable spectroscopic method for multicomponent quantitative analysis of targeted compounds with overlapping signals in complex mixtures has been established. The innovative analytical approach is based on the preliminary chemometric extraction of qualitative and quantitative information from UV-vis and IR spectral profiles of a calibration system using independent component analysis (ICA). Using this quantitative model and ICA resolution results of spectral profiling of "unknown" model mixtures, the absolute analyte concentrations in multicomponent mixtures and authentic samples were then calculated without reference solutions. Good recoveries generally between 95% and 105% were obtained. The method can be applied to any spectroscopic data that obey the Beer-Lambert-Bouguer law. The proposed method was tested on analysis of vitamins and caffeine in energy drinks and aromatic hydrocarbons in motor fuel with 10% error. The results demonstrated that the proposed method is a promising tool for rapid simultaneous multicomponent analysis in the case of spectral overlap and the absence/inaccessibility of reference materials.
Decoding the encoding of functional brain networks: An fMRI classification comparison of non-negative matrix factorization (NMF), independent component analysis (ICA), and sparse coding algorithms.[Pubmed:28322859]
J Neurosci Methods. 2017 Apr 15;282:81-94.
BACKGROUND: Brain networks in fMRI are typically identified using spatial independent component analysis (ICA), yet other mathematical constraints provide alternate biologically-plausible frameworks for generating brain networks. Non-negative matrix factorization (NMF) would suppress negative BOLD signal by enforcing positivity. Spatial sparse coding algorithms (L1 Regularized Learning and K-SVD) would impose local specialization and a discouragement of multitasking, where the total observed activity in a single voxel originates from a restricted number of possible brain networks. NEW METHOD: The assumptions of independence, positivity, and sparsity to encode task-related brain networks are compared; the resulting brain networks within scan for different constraints are used as basis functions to encode observed functional activity. These encodings are then decoded using machine learning, by using the time series weights to predict within scan whether a subject is viewing a video, listening to an audio cue, or at rest, in 304 fMRI scans from 51 subjects. RESULTS AND COMPARISON WITH EXISTING METHOD: The sparse coding algorithm of L1 Regularized Learning outperformed 4 variations of ICA (p<0.001) for predicting the task being performed within each scan using artifact-cleaned components. The NMF algorithms, which suppressed negative BOLD signal, had the poorest accuracy compared to the ICA and sparse coding algorithms. Holding constant the effect of the extraction algorithm, encodings using sparser spatial networks (containing more zero-valued voxels) had higher classification accuracy (p<0.001). Lower classification accuracy occurred when the extracted spatial maps contained more CSF regions (p<0.001). CONCLUSION: The success of sparse coding algorithms suggests that algorithms which enforce sparsity, discourage multitasking, and promote local specialization may capture better the underlying source processes than those which allow inexhaustible local processes such as ICA. Negative BOLD signal may capture task-related activations.
Identifying dynamic functional connectivity biomarkers using GIG-ICA: Application to schizophrenia, schizoaffective disorder, and psychotic bipolar disorder.[Pubmed:28294459]
Hum Brain Mapp. 2017 May;38(5):2683-2708.
Functional magnetic resonance imaging (fMRI) studies have shown altered brain dynamic functional connectivity (DFC) in mental disorders. Here, we aim to explore DFC across a spectrum of symptomatically-related disorders including bipolar disorder with psychosis (BPP), schizoaffective disorder (SAD), and schizophrenia (SZ). We introduce a group information guided independent component analysis procedure to estimate both group-level and subject-specific connectivity states from DFC. Using resting-state fMRI data of 238 healthy controls (HCs), 140 BPP, 132 SAD, and 113 SZ patients, we identified measures differentiating groups from the whole-brain DFC and traditional static functional connectivity (SFC), separately. Results show that DFC provided more informative measures than SFC. Diagnosis-related connectivity states were evident using DFC analysis. For the dominant state consistent across groups, we found 22 instances of hypoconnectivity (with decreasing trends from HC to BPP to SAD to SZ) mainly involving post-central, frontal, and cerebellar cortices as well as 34 examples of hyperconnectivity (with increasing trends HC through SZ) primarily involving thalamus and temporal cortices. Hypoconnectivities/hyperconnectivities also showed negative/positive correlations, respectively, with clinical symptom scores. Specifically, hypoconnectivities linking postcentral and frontal gyri were significantly negatively correlated with the PANSS positive/negative scores. For frontal connectivities, BPP resembled HC while SAD and SZ were more similar. Three connectivities involving the left cerebellar crus differentiated SZ from other groups and one connection linking frontal and fusiform cortices showed a SAD-unique change. In summary, our method is promising for assessing DFC and may yield imaging biomarkers for quantifying the dimension of psychosis. Hum Brain Mapp 38:2683-2708, 2017. (c) 2017 Wiley Periodicals, Inc.
The new KCNQ2 activator 4-Chlor-N-(6-chlor-pyridin-3-yl)-benzamid displays anticonvulsant potential.[Pubmed:23176257]
Br J Pharmacol. 2013 Mar;168(5):1182-200.
BACKGROUND AND PURPOSE: KCNQ2-5 channels are voltage-gated potassium channels that regulate neuronal excitability and represent suitable targets for the treatment of hyperexcitability disorders. The effect of Chlor-N-(6-chlor-pyridin-3-yl)-benzamid was tested on KCNQ subtypes for its ability to alter neuronal excitability and for its anticonvulsant potential. EXPERIMENTAL APPROACH: The effect of 4-Chlor-N-(6-chlor-pyridin-3-yl)-benzamid was evaluated using whole-cell voltage-clamp recordings from CHO cells and Xenopus laevis oocytes expressing different types of KCNQ channels. Epileptiform afterdischarges were recorded in fully amygdala-kindled rats in vivo. Neuronal excitability was assessed using field potential and whole cell recording in rat hippocampus in vitro. KEY RESULTS: 4-Chlor-N-(6-chlor-pyridin-3-yl)-benzamid caused a hyperpolarizing shift of the activation curve and a pronounced slowing of deactivation in KCNQ2-mediated currents, whereas KCNQ3/5 heteromers remained unaffected. The effect was also apparent in the Retigabine-insensitive mutant KCNQ2-W236L. In fully amygdala-kindled rats, it elevated the threshold for induction of afterdischarges and reduced seizure severity and duration. In hippocampal CA1 cells, 4-Chlor-N-(6-chlor-pyridin-3-yl)-benzamid strongly damped neuronal excitability caused by a membrane hyperpolarization and a decrease in membrane resistance and induced an increase of the somatic resonance frequency on the single cell level, whereas synaptic transmission was unaffected. On the network level, 4-Chlor-N-(6-chlor-pyridin-3-yl)-benzamid caused a significant reduction of gamma and theta oscillation peak power, with no significant change in oscillation frequency. CONCLUSION AND IMPLICATIONS: Our data indicate that 4-Chlor-N-(6-chlor-pyridin-3-yl)-benzamid is a potent KCNQ activator with a selectivity for KCNQ2 containing channels. It strongly reduces neuronal excitability and displays anticonvulsant activity in vivo.